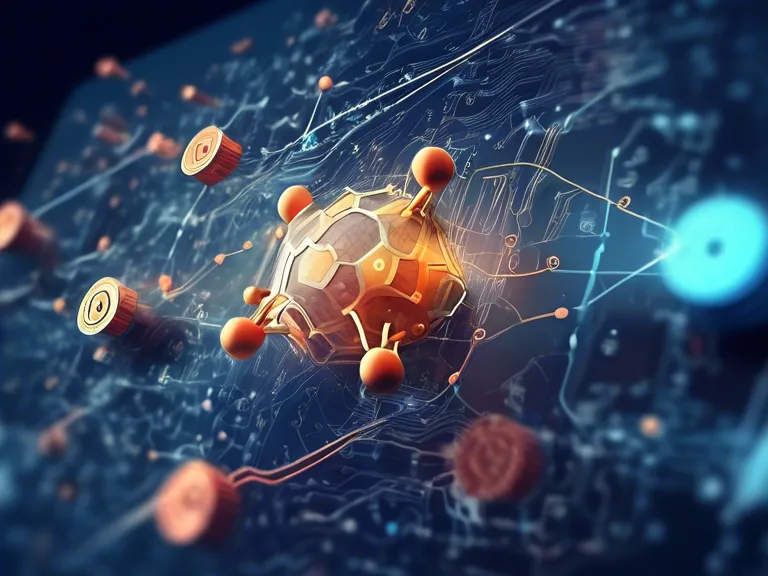
Introduction
Machine learning has revolutionized various industries by enabling automated analysis and prediction tasks. One area where machine learning has shown significant impact is in deposit analysis within the banking and finance sector. By leveraging advanced algorithms and data processing techniques, machine learning models can provide valuable insights into deposit trends, customer behavior, and risk assessment. In this article, we will explore the applications of machine learning in deposit analysis and how it can benefit financial institutions.
Understanding Deposit Analysis
Deposit analysis involves examining the deposit behavior of customers, identifying patterns, and making predictions based on historical data. Financial institutions use deposit analysis to optimize their deposit products, attract new customers, and manage risks effectively. Traditional methods of deposit analysis rely on manual data processing and statistical techniques, which can be time-consuming and limited in scope. Machine learning offers a more efficient and accurate approach to analyze large volumes of data and extract actionable insights.
Machine Learning Applications in Deposit Analysis
Customer Segmentation
One key application of machine learning in deposit analysis is customer segmentation. By clustering customers based on their deposit behavior, demographic information, and transaction history, financial institutions can tailor their marketing strategies and product offerings to specific customer segments. Machine learning algorithms such as clustering, classification, and regression can help identify similar groups of customers and predict their deposit preferences.
Churn Prediction
Predicting customer churn is crucial for banks to retain existing customers and prevent deposit attrition. Machine learning models can analyze historical data to identify patterns that indicate potential churn behavior. By analyzing factors such as account activity, transaction frequency, and customer interactions, machine learning algorithms can predict which customers are likely to close their accounts or reduce their deposits. This allows banks to take proactive measures to retain customers and improve customer satisfaction.
Fraud Detection
Detecting fraudulent activities in deposit transactions is a critical task for financial institutions to protect against financial losses and maintain trust with customers. Machine learning models can analyze transaction data in real-time to detect anomalies and flag suspicious activities for further investigation. By leveraging techniques such as anomaly detection, pattern recognition, and predictive modeling, machine learning can enhance fraud detection capabilities and improve the overall security of deposit accounts.
Risk Assessment
Machine learning can also assist in risk assessment for deposit products by analyzing customer creditworthiness, loan repayment history, and financial stability. By building predictive models that assess the likelihood of default or delinquency, financial institutions can make informed decisions on offering deposit products to customers. Machine learning algorithms such as decision trees, random forests, and neural networks can help automate the risk assessment process and improve the accuracy of credit scoring models.
Conclusion
Machine learning applications in deposit analysis offer a powerful tool for financial institutions to gain insights into customer behavior, manage risks, and enhance operational efficiency. By leveraging advanced algorithms and data processing techniques, banks can optimize their deposit products, improve customer retention, and detect fraudulent activities more effectively. As the financial industry continues to embrace digital transformation, machine learning will play a crucial role in shaping the future of deposit analysis and driving innovation in banking services.