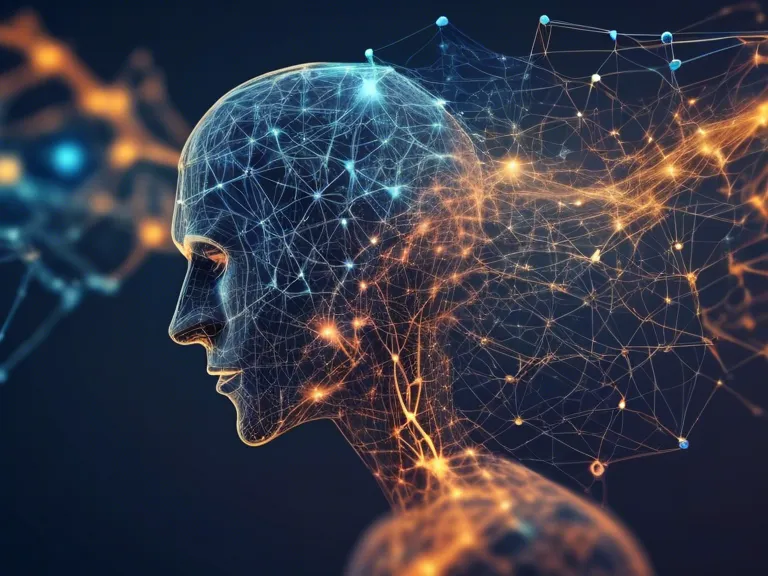
Introduction
Machine learning has become an indispensable tool for optimizing various business processes, including evaluating the potential of aged domains for resale. In the realm of digital marketing, the practice of "jual" (selling) aged domains is a lucrative business opportunity that can be greatly enhanced through the application of machine learning techniques. By leveraging machine learning algorithms, marketers can analyze large volumes of data to identify valuable aged domains, predict their potential resale value, and make more informed decisions.
Understanding Aged Domains
Aged domains refer to domain names that have been registered for an extended period, ranging from several months to many years. These domains often have a history of established online presence, accumulated backlinks, and potential for organic traffic. Marketers interested in jual aged domains seek out these properties for their potential to rank well in search engines and attract valuable traffic.
Leveraging Machine Learning for Domain Evaluation
Data Collection and Preprocessing
The first step in optimizing the potential of jual aged domains through machine learning is collecting relevant data. This may include domain age, backlink profile, traffic metrics, content quality, and historical performance. Once the data is gathered, it needs to be preprocessed to ensure accuracy and consistency before feeding it into machine learning algorithms.
Feature Selection and Engineering
Feature selection plays a crucial role in the success of machine learning models for domain evaluation. By identifying the most relevant features that influence the resale potential of aged domains, marketers can create predictive models that are more accurate and efficient. Feature engineering techniques can also be applied to derive new features or transform existing ones for better model performance.
Model Training and Evaluation
Machine learning models can be trained using various algorithms such as regression, classification, or clustering, depending on the specific goals of domain evaluation. These models are then evaluated using metrics such as accuracy, precision, recall, and F1 score to assess their performance and fine-tune them for optimal results. Cross-validation techniques can also be employed to ensure the robustness of the models.
Predicting Resale Value
One of the key objectives of applying machine learning to jual aged domains is predicting their resale value accurately. By training regression models on historical domain sales data and relevant features, marketers can forecast the potential resale value of aged domains with a high degree of confidence. This enables them to make informed decisions on pricing and negotiation strategies.
Conclusion
Mastering machine learning for optimizing jual aged domain potential can provide marketers with a competitive edge in the dynamic domain resale market. By leveraging advanced algorithms, data analytics, and predictive modeling techniques, marketers can unlock valuable insights, identify profitable opportunities, and maximize the returns on their investment in aged domains. As machine learning continues to evolve, its role in domain evaluation and optimization is expected to become even more critical for success in the digital marketing landscape.